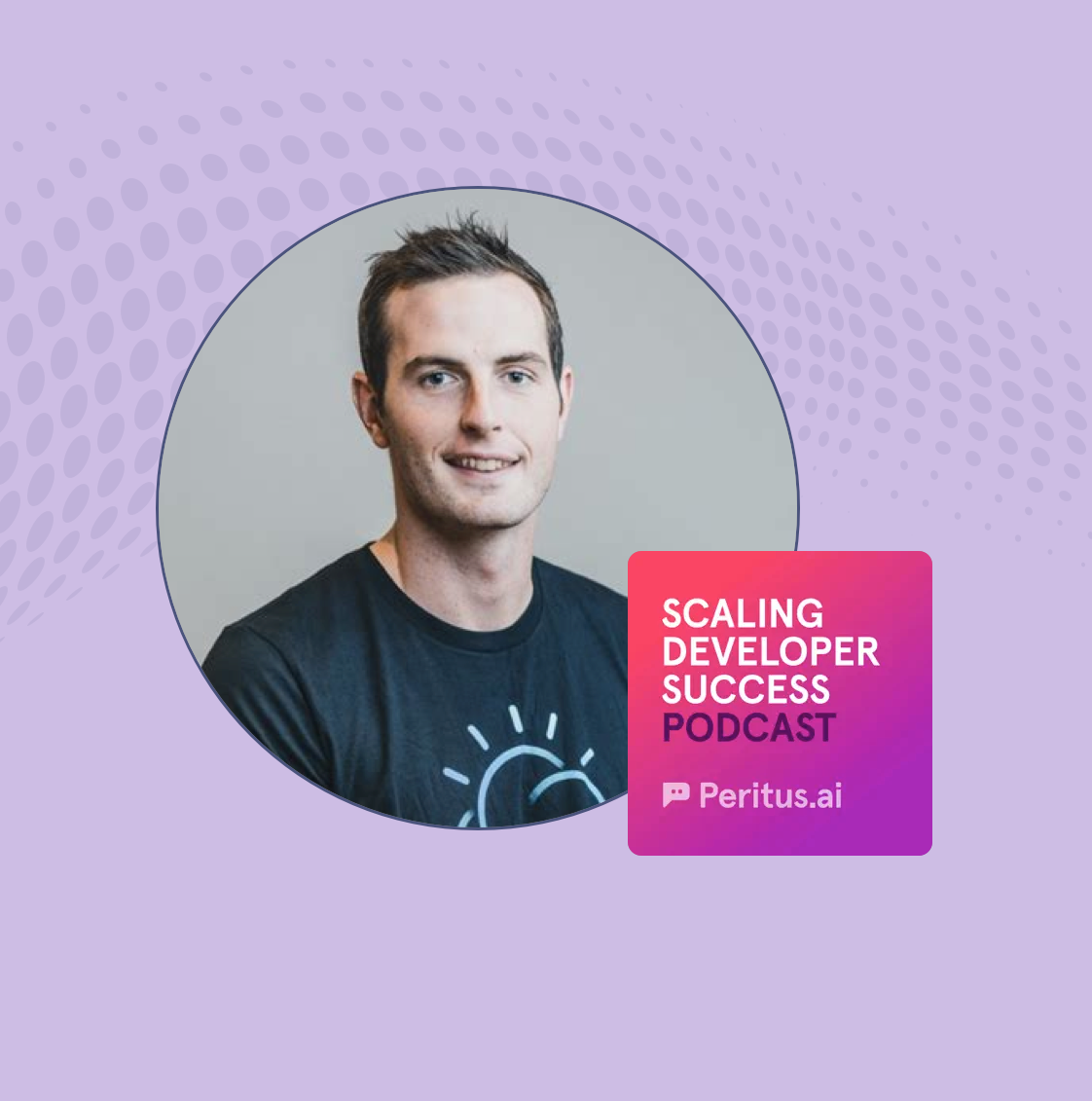
DevRel Leader Spotlight - Ed Shee, Seldon
Peritus.ai
May 13, 2022
As Machine Learning (ML) models become ubiquitous across enterprise software solutions, developer education and enablement occupies an increasingly central role in building the next generation of AI applications. In this podcast, Pertius CEO Robin Purohit explores the challenges and opportunities within the space of ML operations with Ed Shee, Head of Developer Relations at Seldon.
The Advent of ML Operations
When Ed Shee laid the foundations of his career at IBM, DevOps and Data Science represented distinct operational functions, independent from Machine Learning. Towards the end of his tenure at IBM, however, the field of ML Operations began emerging.ML Ops lies at the intersection of DevOps, Machine Learning and Data Science. Rather than focusing on optimal processes for deploying software stacks, or building models, ML Ops addresses challenges within the rapidly expanding space of ML applications. The line of inquiry poses questions such as: How do you move a massive quantity of ML models into production while navigating the demanding expectations of business stakeholders? How do you integrate those models into existing business systems? And, where do you find talent that understands the world of DevOps as well as the Data Science discipline required for model development and building statistical algorithms? Shee cut his teeth on these challenges for X years, while leading a team of engineers and developer advocates at IBM.
The Proliferation of DevRel & ML Ops
The growth of ML Ops led to an explosion of new tools, companies, and platforms dedicated exclusively to ML engineers. Only a few months ago, most vendors failed to permit engineers to build ‘feature stores,’ or repositories for storing or retrieving patterns within their datasets. Virtually overnight, an entire landscape of vendors surfaced offering exactly that.Today, Shee and his DevRel team at Seldon focus specifically on educating ML engineers on best practices, reading through the noise, and identifying combinations of tools and repositories optimized for their needs. Ultimately, the flurry of innovation in ML Ops has propelled the need for evolution of DevRel into its own dedicated function within the enterprise.
An Alliance among DevRel and Product Teams
Shee reports directly to the VP of Product at Seldon, and has built a strong alliance with the entire Product team to support DevRel. “I think the whole reason DevReal exists is to make it easier for people to use your products,” he explains. At earlier stages in his career, his role was siloed, lacked departmental backing or horizontal integration, and he struggled to gather, assess and implement user feedback. Absent a network of product managers, that hold sway over the development roadmap, prioritizing product refinements based on customer engagement proved remarkably challenging. “If I'm hearing stuff in the community… that users and developers are screaming out for a feature… I need a seat at the table when they're discussing what goes into the next release so that I can get it in there,” he explains. Shee leverages his DevRel team at Seldon to channel the voice of the developer and customers, to guide the vision of the Product team, and empower ML engineers.
Where does DevRel Fit within a Company?
Traditional businesses have little need for DevRel teams. Software engineering teams and product teams can manage customer feedback from users in less technical businesses, while marketing can push the product out to users. The imperative forDevRel emerges in technical businesses that sell developer-focused products and solutions. Traditional outreach channels are less effective in targeting a technical user base that is fundamentally averse to paid advertisements, marketing campaigns or direct sales. Developers tend to prioritize sovereign decision making, and flexible and customized products tailored for their needs. “They don't want to be told this is the tool you should have, and here's why,” Shee explains. Steeped in a scientific mindset, honed by years of skepticism and inquiry, engineers have been brought up to value data driven decisions that leaves them in charge of as many product levers as possible. This keen understanding of Developer personas and preferences is what justifies a DevRel team.
Measuring Success of DevRel
Unabashedly “anti metrics,” Shee believes excessive efforts to ‘measure success’ can do more to drive behavior than to drive results. At IBM, his team was initially driven entirely by the number of cloud signups and user activity KPIs. “We could go to our top enterprise customer and run a workshop with their influential developers,” he recalls, “and if we only got 10 people to sign up to the cloud platform, we would have been better off running a workshop with 300 students.” Measuring the success of DevRel exclusively through a metrics-only approach runs the risk of losing qualitative factors or choosing the wrong KPI in an ambiguous field. At Seldon, Shee has developed a more granular approach to gauging the success of his team. He looks at the usage and adoption of each open-source library on Github, the number of messages and activity in their associated Slack communities, and even the number of questions the developers in those communities ask each month. He then ties these data points to the efficacy of their developer-facing documentation, as more effective documentation should result in fewer questions. It’s important to maintain a macro lens to see how data, usage, behavior, and metrics interact to evaluate the success of DevRel initiatives.
The Right Channels to Reach Developers
When selecting appropriate channels to reach developers, Shee and his team carefully considered Slack, Reddit, and Discord, amongst others. If Seldon only offered a single API as the product, then Shee suggests that Stack Overflow would qualify as the optimal channel, since many developers would likely ask recurring questions. Slack, however, generates higher engagement and success rates, and even cultivates a sense of community, as independent members often engage with one another directly, in real-time (or even asynchronously) to great effect. Rather than Stack Overflow, which was ‘black-and-white’, Slack offered an organic community to help developers address nuanced problems which might have the same parameters as someone else’s challenge. Seldon also runs bi-weekly community calls where they provide product updates, roadmaps, and general thoughts about the open-source community. User feedback, on feature preferences or adoption hurdles, is encouraged and celebrated, leading to a robust, effective and engaged developer ecosystem.
DevRel: A Culture Shift
Shee sees a bright future for DevRel. Over the last 20 years, all businesses have become technology companies, he remarks. Digital transformation initiatives, upskilling employees and reorganizing horizontal functions, only serves to accelerate the adoption of tech-first skill sets. In turn, he observes, new challenges and hurdles emerge spinning the flywheel faster and faster, especially once ML Ops are introduced into the equation. The growth of DevRel in businesses selling developer-focused tools is manifest. However, DevRel in less technically oriented product companies will not escape the wave, he believes. In these companies, DevRel takes on the characteristics of HR, with a mix Public Relations and a more technical focus. In all scenarios, he predicts, DevRel will continue to evolve, and will inevitably become more interdisciplinary and far-reaching across the enterprises of the future.